Interactive Video Stylization Using Few-Shot Patch-Based Training
ACM Transactions on Graphics (Proceedings of SIGGRAPH 2020) 39(4):73, 2020
In this paper, we present a learning-based method to the keyframe-based video stylization that allows an artist to propagate the style from a few selected keyframes to the rest of the sequence. Its key advantage is that the resulting stylization is semantically meaningful, i.e., specific parts of moving objects are stylized according to the artist s intention. In contrast to previous style transfer techniques, our approach does not require any lengthy pre-training process nor a large training dataset. We demonstrate how to train an appearance translation network from scratch using only a few stylized exemplars while implicitly preserving temporal consistency. This leads to a video stylization framework that supports real-time inference, parallel processing, and random access to an arbitrary output frame. It can also merge the content from multiple keyframes without the need to perform an explicit blending operation.We demonstrate its practical utility in various interactive scenarios, where the user paints over a selected keyframe and sees her style transferred to an existing recorded sequence or a live video stream.

Best in Show Award at SIGGRAPH Real-Time Live!
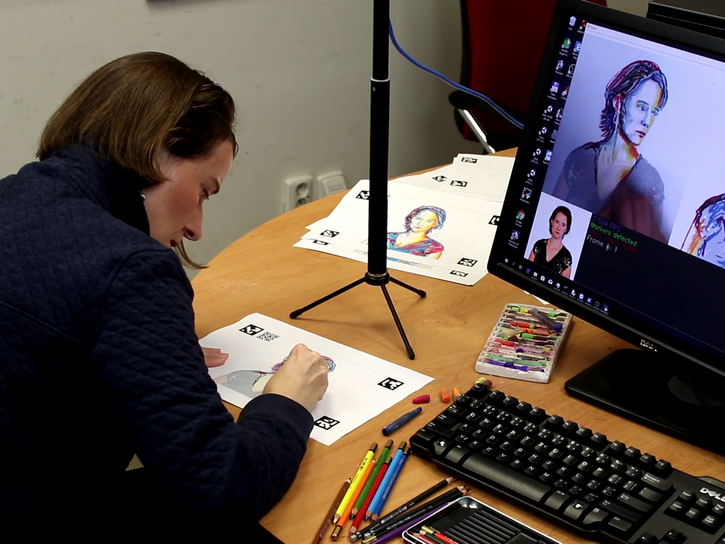